Platform & Services
Layer 3 – HYPERGRYD Integrated Platform and Services
Development of an overall platform-of-platforms, which offers seamless transition between different specialized platforms to monitor, analyze and operate the whole system from different aspects and different life stages. Several important services will be developed within the HYPERGRYD project, based on the tools
developed in Layer 2, but external services will also be able to interface directly with the platform through an open API.
Novel Machine Learning (ML) methods for facilitating coupled electric and thermal load forecasting
HYPERGRYD will develop a set of data analysis and machine learning tools and models for the purpose of forecasting energy generation and demand in DHC networks, based on different parameters for both heat and electricity, up to 24 hours in advance.
Anomaly detection and predictive maintenance
Using advanced data analytics and Artificial Intelligence (AI), this service will be capable of anomaly detection and suggesting preventive maintenance actions in DHC supply and distribution. It will alert the end-user and use GIS and BIM to attempt to locate the problem in the Digital Twin, to assist further inspection.
Demand Response management and predictive control
A Demand Response management service will be developed to optimally balance the utilization of different energy carriers (thermal and electricity) in real-time, taking into account both locally available DERs (renewables, storages, heat pumps) and grid resources (electric and DH).
Market-aware Demand Response management with self-adapting trading strategies
HYPERGRYD will foster Demand Response methods that can operate on real-time energy markets, with dynamic energy prices through methods from Artificial Intelligence (AI) and Machine Learning (ML)/data analysis, such as reinforcement learning, predictive control, and forecasting. This service will expose an open API to interface with hardware, grid operators and utilities, or could also be deployed on an edge device.
Enhanced smart edge computing techniques, leveraging IoT and distributed learning
HYPERGRYD will develop methods for edge-based distributed machine learning and control, which allows ML models and control systems to be distributed across multiple edge devices and geographic locations, for joint modelling, control, and optimization. This will lead to reductions in latency, improved reliability (important for real-time control for grid applications), and reduced
bandwidth usage.
Advanced visualization and easy-to-use functionalities with end-user engagement
A service for continuous monitoring of system parameters (e.g., from temperature and pressure sensors in valves), and visualization of the above services will be developed. Users will be able to create and schedule maintenance tasks, and access predictive maintenance reports generated by the AI (Key Enabling Service 2). This will allow for highly visual remote monitoring and simplify the process of scheduling maintenance trips and tasks on site. HYPERGRYD develops the visualized end-user engaging services to put in place proper collaboration schemes between the end users and the utilities (CHP operators and electrical grids), this way, becoming an active player able to provide balancing services.
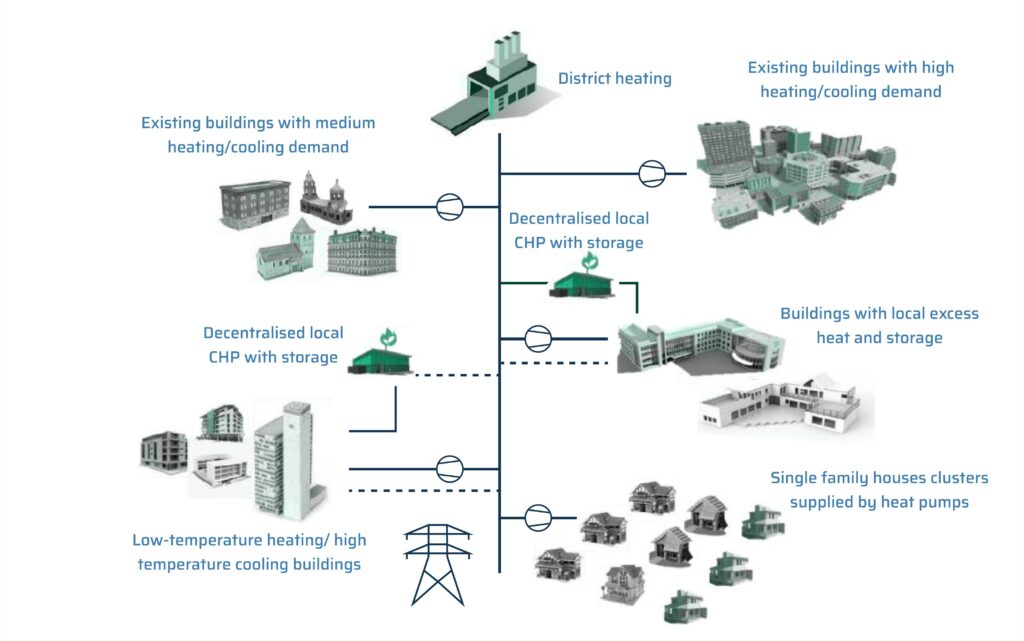